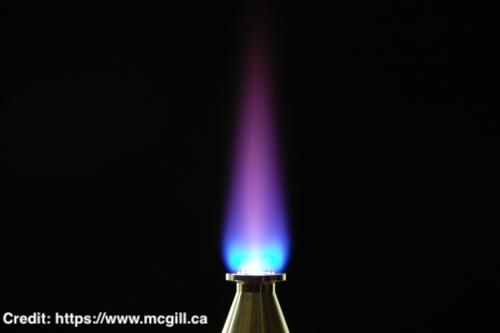
New technological challenges in combustion science require reliable ignition and flame stabilization in demanding conditions. Some examples are ultra-lean combustion, fuel flexibility (using alternative, low-carbon fuels), supersonic combustion (scramjets), and the active control of thermo-acoustic instabilities. Non-thermal plasma discharges have been proposed as an innovative solution to ensure efficient and stable operation in these particular regimes. Nanosecond discharges are introduced to obtain a more favourable ignition of reactive mixtures, where conventional methods fail. However, an in-depth knowledge of the effects of non-equilibrium plasma on the initiation and stability of these challenging combustion processes is still lacking in the field. The current literature on the subject is incomplete and mostly experimental. The modelling and numerical simulation of plasma discharges and their influence on combustion therefore remains a critical need to understand and support experiments working towards the development of the next combustion technologies.
The numerical simulation of plasma-assisted combustion (PAC) problems remains a key challenge in the community because of (1) the multi-scale nature of the flow: plasma chemistry occurs at the nanosecond time scale and combustion at millisecond scales; (2) non-equilibrium effects: combustion chemistry and transport are coupled with detailed plasma chemistry in non-equilibrium thermodynamics; and (3) the large dimensionality of the mechanisms: hundreds of species are coupled tightly in large and stiff kinetic mechanisms. It is therefore key to develop reliable reduced-order representations of the detailed chemistry in order to alleviate the aforementioned numerical challenges.
The overall goal of the proposed research is to fill the existing gaps in the numerical modelling of PAC by proposing a comprehensive numerical study on the plasma-assisted ignition and combustion of fuel-air mixtures by nanosecond pulse discharges using reliable reduced-order models. Our reduction tools are based on (1) data-driven methods as principal component analysis (PCA) in combination with machine-learning algorithms (ANN, GPR); and (2) novel graph-based techniques such as DRGEP.
Our partners in this project are the research group of professor Bisetti at the University of Texas at Austin (USA) and professor Pepiot at Cornell University (USA).